Projects
Efficient Communication Techniques for Federated Edge Learning over Wireless Networks
Project description/goals
Pushing artificial intelligence from cloud to network edge to realize edge intelligence is an important vision in 6G communications. By distributing training across edge devices and aggregating local models in edge server, federated edge learning can make full use of distributed data for model training while preserving the data privacy, which is regarded as a key enabling technology for edge intelligence.
Importance/impact, challenges/pain points
With the explosive growth of intelligent devices, federated edge learning is facing a communication bottleneck that restricts its large-scale expansion: in order to update the global model, the uploading of high-dimensional local models from massive users will bring a great burden to the air interface and lead to serious communication delay.
Solution description
In order to overcome the communication bottleneck and improve the scalability of federated edge learning, this project proposes an innovative scalable technology, called over-the-air computation (AirComp), with the main feature of communication-computation integration. By AirComp, the desired model aggregation is performed over the air via exploiting the wave-superposition property of multi-access wireless propagation. The approach breaks the limitation of the classic design principle of decoupling communication and computation, and fundamentally solve the communication bottleneck.
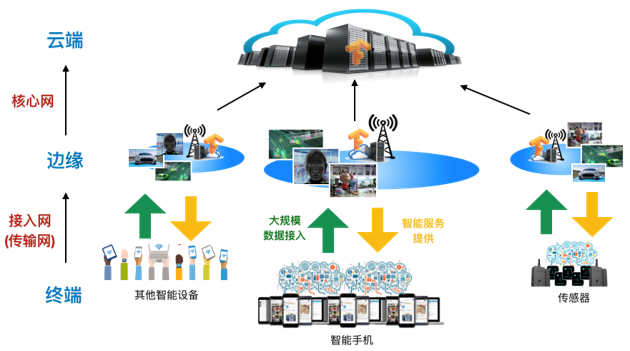
Key contribution/commercial implication
This project aims to develop a comprehensive communication-efficient solution framework for large-scale federated edge learning that consists of system design, resource allocation and performance analysis. The solution framework is supposed to open up a new design paradigm for edge learning network featuring communication-computation integration.
Next steps
To address the communication bottleneck of large-scale federated edge learning, the next step of this project focuses on the following three main contents as planned: 1) system design based on communication and computing integration, 2) end-to-end edge learning performance analysis, and 3) wireless resource adaptation for edge learning network. Finally, a scalable and communication-efficient solution for federated edge learning will be developed.
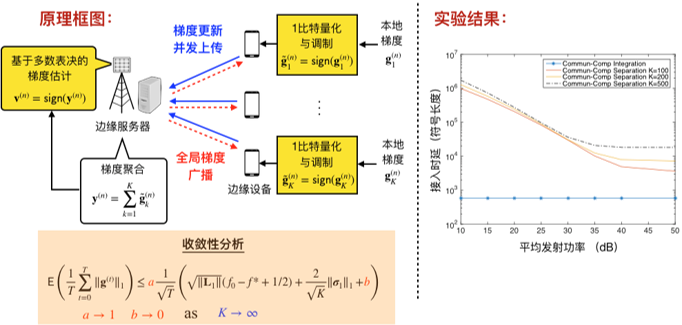
Collaborators/partners
None.
Team/contributors
Guangxu Zhu.