AI Assisted Optimization for Radio Resource Management in Large-Scale Wireless Networks
Project description/goals
As 6G wireless networks are shifting to the new paradigm of global coverage, all spectra, and full applications, efficient radio resource management becomes the key to release the potential of 6G systems. Nevertheless, the large-scale radio resource management is made highly complex by high connection density, extremely heterogeneous networks, and the interference in diverse communication scenarios. Conventional optimization methods limit their applications for solving large-scale problems in real time due to the iterative algorithm framework. In contrast, AI assisted optimization can largely reduce the computational burden as it avoids the repetitive solving process on a case-by-case basis by utilizing historical experience on the same type of problems.
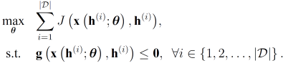
Importance/impact, challenges/pain points
AI assisted optimization for large-scale radio resource management involves new problems in three aspects: 1) how to satisfy complex constraints; 2) how to scale and generalize to large-scale problems; 3) how to adapt to dynamic environment.
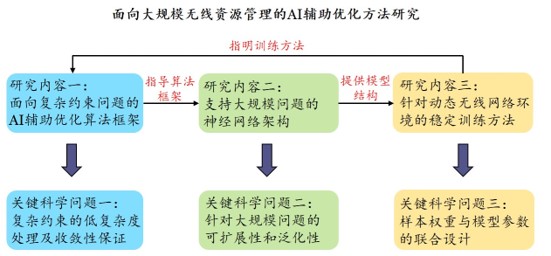
Solution description
This project explores the combination of AI techniques and the theories and methods of optimization, aiming for developing an AI assisted optimization algorithm framework for solving complex constrained problems, designing a neural network architecture for tackling large-scale problems, and constructing a stable training method in dynamic environment.
Key contribution/commercial implication
This project aims for exploring the combination of AI techniques and optimization theories, providing a novel method for large-scale radio resource management. The proposed paradigm of AI assisted optimization is hoped to boost the potential of 6G large-scale wireless networks.
Next steps
1) Develop an AI assisted optimization algorithm framework for solving complex constrained problems. 2) Design a neural network architecture for tackling large-scale problems. 3) Construct a stable training method in dynamic environment.
Collaborators/partners
N/A
Team/contributors
LI Yang