Academic Exchange
Dr. Pu Wenqiang: The Spark Between Academic Research and Engineering Practice
Dr. Pu Wenqiang currently serves as a Research Scientist at the Shenzhen Research Institute of Big Data. He obtained both his bachelor's degree and Ph.D. in engineering from Xi’an University of Electronic Science and Technology in 2013 and 2018, respectively. From January 2015 to September 2018, he conducted research as a visiting Ph.D. student at the Chinese University of Hong Kong, Shenzhen, and engaged in postdoctoral research there from January 2019 to September 2020. Since joining the Shenzhen Big Data Research Institute in October 2020, Dr. Pu has focused on large-scale signal processing, sensor network resource scheduling, first-order optimization methods, and intelligent game theory. He has published several papers in authoritative international journals and conferences in the field of signal processing and currently leads a National Natural Science Foundation Youth Project as well as multiple industry projects.
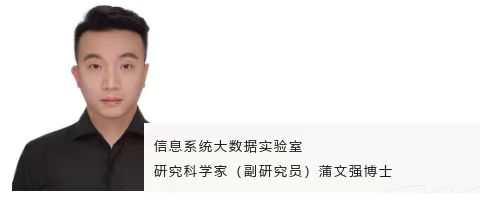
Dr. Pu began exploring the combination of “academia” and “engineering” during his student years. While pursuing his Ph.D. at Xi’an University of Electronic Science and Technology, he spent considerable time collaborating with the Chinese University of Hong Kong, Shenzhen, participating in the research efforts of two distinctly styled research groups. The group at Xi’an University was primarily focused on research directed at engineering practices and emphasized building students’ abilities to establish physical concepts for engineering problems. In contrast, the group at the Chinese University of Hong Kong, Shenzhen, concentrated on mathematical optimization and aimed to cultivate a solid mathematical foundation and understanding of mathematical tools among students. Through his exchanges and research work in these two different environments, Dr. Pu successfully integrated the knowledge and skills he gained from both academia and engineering, fostering his ability to apply academic methods and mathematical optimization in practical engineering scenarios.
During his Ph.D. studies, Dr. Pu experienced a year-long adjustment period to find a balance between academia and engineering. “Initially, my research was a trial-and-error process,” he noted. “Throughout my research journey, I discovered that the industry typically focuses solely on engineering problems, while academia tends to concentrate on theoretical foundations. It’s not easy to truly combine these two aspects.”
After leading several collaborative projects with industry during his doctoral studies, Dr. Pu realized that a clear understanding of physical concepts is crucial to solving engineering problems. The correctness of these concepts determines the effectiveness of the mathematical tools employed. However, even when identifying an entry point to a problem, lacking appropriate mathematical tools means the problem cannot be fully resolved. Finding an organic combination of engineering and research is essential for realizing practical application value. To continue pursuing his “academic × engineering” research philosophy, Dr. Pu chose to remain at the Shenzhen Big Data Research Institute (SRIBD). As a research institute, SRIBD provides a rich environment for integrating research and practice, aligning with Dr. Pu’s research philosophy and aspirations.
In 2015, when SRIBD was still in its early stages, Dr. Luo Zhiquan led a small innovative team in big data research. Dr. Pu became aware of this team at that time and later participated in some of the institute's preparatory work. “During my doctoral studies, I learned about a powerful big data research team at the Chinese University of Hong Kong, Shenzhen, and I even had the opportunity to join team projects. The experiences I had during this time left a deep impression on me.” Therefore, after completing his postdoctoral research, Dr. Pu officially joined SRIBD as a research scientist in the Information Systems Big Data Laboratory.
Currently, Dr. Pu collaborates with industrial partners on research related to signal processing, involving interdisciplinary applications. He believes there are organic points of connection between academia and engineering. “If you find that the tools from other disciplines do not fully apply to industrial problems, you need to research new theories and methods that fit the specific boundary conditions of the engineering problem.”
Q&A with Dr. Pu
Q: What kind of problems do you prefer to explore?**
A: I personally enjoy interdisciplinary learning and acquiring new tools. I also hope to extract key methods driven by application scenarios to solve strong application needs. Many people who work with tools may not understand engineering well, while engineers may not have a deep understanding of mathematics, leading to a disconnect. Our research institute focuses on cutting-edge issues, and by solving these problems, we can experiment with industry applications. What we aim to do is connect academia and industry to create relevant links.
Q: With the explosive growth of information technology, how have research directions in signal processing changed?**
A: Signal processing is a discipline that combines applied mathematics and systems theory. "Signals" can be defined as any physical quantity that transmits information, such as sound, images, time series data, and biomedical measurements. Signal processing has applications in various fields, including communications, audio and video processing, biomedical engineering, seismic analysis, finance and economics, image processing, and computer vision. Over the past decade, advancements in computational power and the growth of data have led to increasing intersections between signal processing and other fields (such as machine learning and data science), opening up many new research and application opportunities.
Q: What was your initial motivation for engaging in scientific research?**
A: I didn’t start with the goal of solving a world problem. Instead, I discovered various issues or areas where I could contribute ideas that I was interested in, and I continued to pursue those.
Q: Is it difficult to identify problems?**
A: It depends on how you define it. One form of innovation occurs because no one else has tackled the problem, while another arises because you realize its importance and want to solve it. In fact, for many engineering problems, understanding what the issue is—whether it’s a physical mechanism problem or a mathematical tool problem—solves about 70% of the challenge.
Q: How do you spend your time outside of research?**
A:** In my free time, I like to learn more about other disciplines. With the rise of social media, I enjoy watching videos from content creators that interest me.